N1-0344 (D) Machine learning algorithms in data intensive astrophysics in the age of LSST
In this project we are in collaboration with Hungarian researchers developing and testing machine learning-based Big Data algorithms to support the scientific investigations of the Vera Rubin Observatory’s Legacy Survey of Space and Time (LSST) sky survey project. We are developing image-based and parameter-based, supervised classification algorithms using deep convolutional networks for the classification of LSST variable star data (CSFK). The difficulty so far is the lack of the LSST data, therefore we use ZTF and OGLE data. On the other hand, sparse data have to be used to identify very different timescales of variation. The results will be updated and refined as more and more LSST data become available. We also develop artificial intelligence-based algorithms for the detection and classification of transient phenomena based on simulated LSST data (UNG). In the case of real LSST measurements, we assemble a database of transient light curves that will help to select and analyse the most interesting objects. We are also developing image-based artificial intelligence algorithms for the morphological analysis of the Solar system objects, mainly comets: the aim is to identify fine structures and active regions and to perform tail-coma separation (ELTE GAO). Here, SDSS and ZTF data will provide the training sample. The uniqueness of the Slovenian-Hungarian project, besides the complementary and synergistic nature of the participating research teams, is that all three subtasks are related to the preparation of the LSST, all three use LSST proxy data, and all three aim at developing state-of-the-art machine learning based algorithms, where partners mutually support each other in solving similar subtasks.
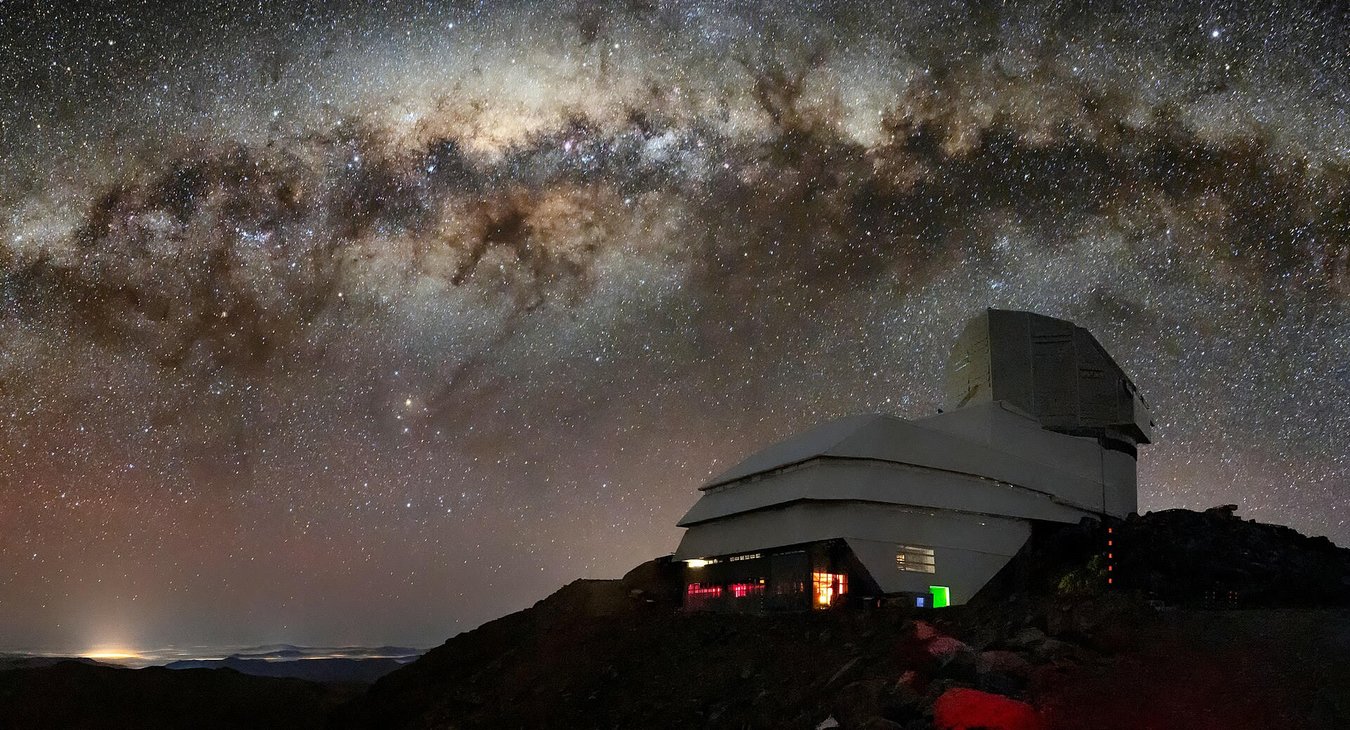